La logica del sistema: azione suggerite dallo Smart Agent
Risultati ottenuti
Il sistema, grazie alla logica di reinforcement learning, migliora progressivamente partendo dalla base di conoscenza costruita dagli esperti. Questo trasferimento di conoscenza ha reso il sistema, in pochissimo tempo, autonomo e pressoché infallibile.
Questi risultati sono infine confluiti in una pubblicazione scientifica dal titolo “A decision theory approach to support action plans in cooker hoods manufacturing”
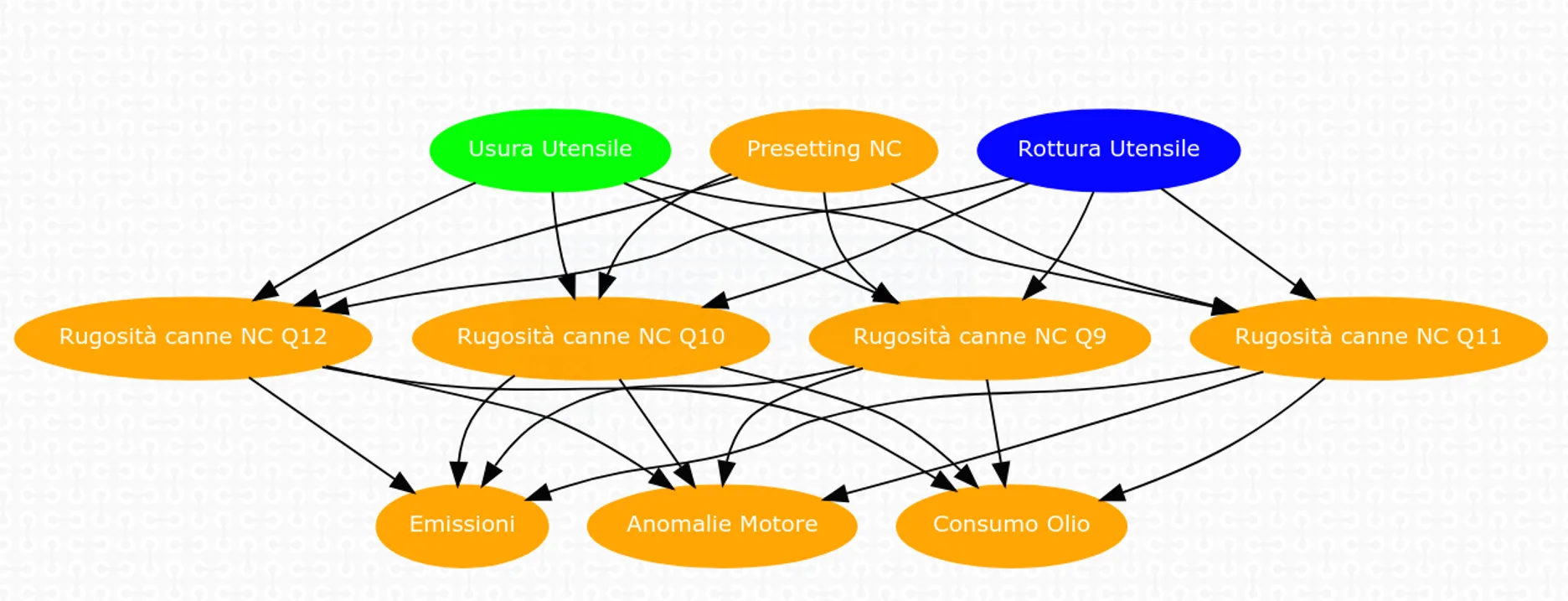
Implementazione della Rete Bayesiana per la Gestione Probabilistica dei Problemi di Qualità
Il primo passo nell'implementazione di un sistema A.I.O.C.A.P. è creare una base di conoscenza probabilistica.
Questa base, organizzata in una rete Bayesiana, consente al sistema di proporre soluzioni sotto forma di action plan.
La rete Bayesiana calcola il grado di credenza probabilistica dello stato attuale del processo, in seguito a eventi legati a problemi di qualità rilevati automaticamente dal sistema.
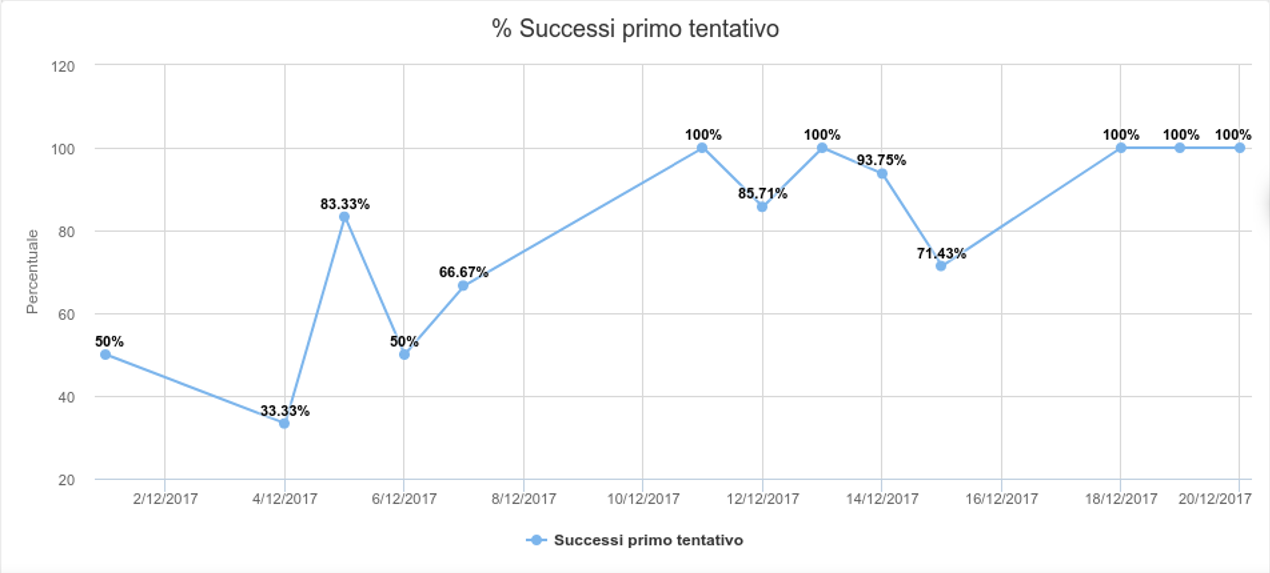
Curva di apprendimento
Dai grafici relativi all’utilizzo presso lo stabilimento di un nostro cliente, si evidenzia come, dopo circa un anno di apprendimento, il sistema abbia registrato una media del 95% di successi al primo tentativo.
Nella prima fase, il grafico mostra una tendenza all'apprendimento, sebbene con qualche fluttuazione. Tuttavia, in pochi mesi, il sistema raggiunge ottimi risultati.
Il valore aggiunto del sistema è confermato dal supporto che fornisce ai nuovi operatori, non esperti del processo produttivo. Il sistema trasferisce la conoscenza appresa dagli operatori esperti, ottimizzando così l’apprendimento e le performance.
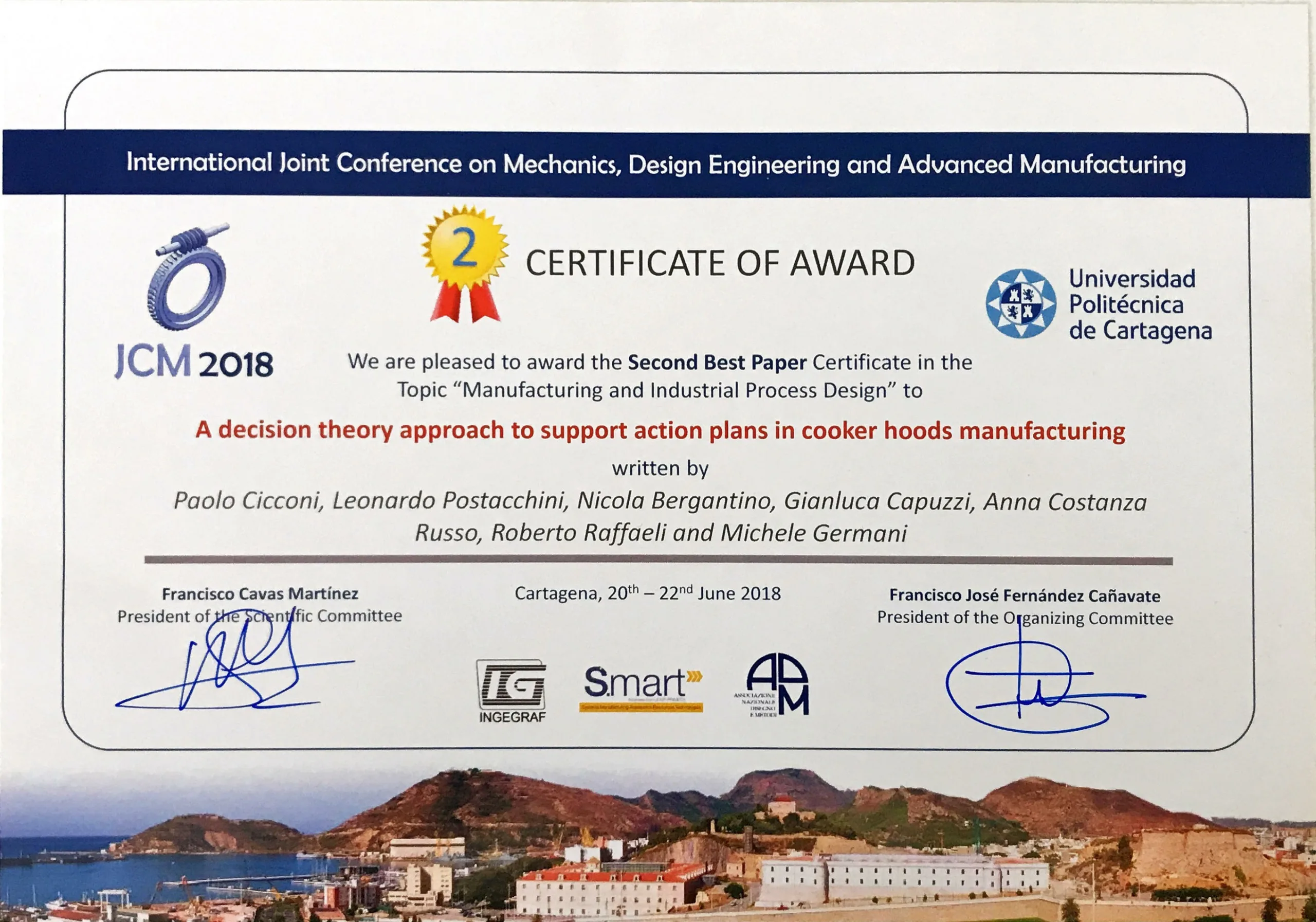
Ottimizzazione dei Processi di Produzione: Un Case Study nel Settore delle Cappe da Cucina
Il progetto ha dato vita a un case study, che è stato alla base di una pubblicazione scientifica vincitrice del riconoscimento di “Second best paper” dall’Università di Cartagena, nell’ambito del tema “Manufacturing and Industrial Process Design”. Il titolo del paper è “A decision theory approach to support action plans in cooker hoods manufacturing”.